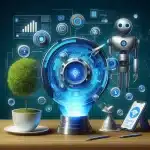
What is AI hallucination and how does it impact marketing?
By Dania Kadi.
Marketers increasingly rely on Artificial Intelligence (AI) as a reliable tool for generating factual information and crafting data-driven strategies. It is particularly useful in digital marketing, creating content, generating calls to action and recommending keywords and hashtags. However, even AI can become delirious , which seems like something from science fiction. One such flaw is AI hallucination—a phenomenon where AI systems produce information that appears convincing but is, in fact, incorrect or entirely fabricated.
AI hallucination can occur when AI models, particularly those used in language processing, generate outputs that are not grounded in reality. This may happen due to biased training data, the model’s limitations, or errors in the algorithm’s interpretation of data.
For marketers, the implications of AI hallucination can be significant:
- Misleading content: AI-generated text may contain errors that misinform audiences.
- Brand reputation: Inaccurate information produced by AI can harm a brand’s credibility.
- Customer trust: Reliance on AI-generated content that turns out to be false can lead to a loss of trust among consumers.
While AI offers powerful tools for marketing, understanding and mitigating the risks of AI hallucination is crucial to maintaining accuracy and trust in brand communications.
Understanding AI hallucinations
The term “AI hallucination” refers to a phenomenon where Artificial Intelligence systems, particularly those using advanced language models, generate information that seems plausible but is actually incorrect, misleading, or entirely fabricated. Unlike human errors, where misinformation might result from misunderstanding or lack of knowledge, AI hallucinations occur despite the system’s seemingly vast and accurate database.
AI models are trained on extensive datasets, absorbing patterns and information to generate responses, recommendations, or content. However, these models don’t truly understand the content they produce; they simply predict what comes next based on the data they’ve processed. When the data is incomplete, biased, or taken out of context, the AI may generate outputs that aren’t grounded in reality. This is what we refer to as hallucination.
For instance, an AI might produce a confidently written marketing message that cites statistics or facts that don’t exist. While the output might look and sound professional, the information could be entirely fabricated, leading to significant risks if used without proper verification.
Understanding AI hallucination is crucial because it highlights the limitations of AI, reminding marketers that while AI can be a powerful tool, it requires careful oversight and validation to ensure the accuracy and integrity of the information it generates.
How does AI hallucination occur?
AI hallucination occurs when an Artificial Intelligence system generates outputs that are factually incorrect, misleading, or entirely fabricated. This phenomenon stems from a few key factors in how an AI driven large language model is developed and functions. You might also come across something called “Lllm hallucination” which happens when a large language model (LLM) produces a response that is factually inaccurate, illogical, or unrelated to the input prompt.
- Training data limitations: AI models are trained on vast datasets, absorbing patterns and associations from the information they process. However, if the training data is biased, incomplete, or contains errors, the AI can produce outputs that reflect these flaws. When an AI model encounters a situation where it lacks adequate data or context, it may “hallucinate” by generating a response that fills in the gaps, often with fabricated or misleading information.
- Overfitting and generalisation errors: Overfitting occurs when an AI model becomes too closely aligned with its training data, learning to replicate specific patterns rather than understanding broader concepts. This can lead to generalisation errors, where the AI applies incorrect patterns to new, unseen data. In such cases, the AI might produce outputs that are inaccurate or nonsensical, appearing to “hallucinate” as it attempts to apply its over-learned patterns inappropriately.
- Lack of true understanding: Despite their sophistication, AI models don’t understand the content they generate. They function by predicting the next word or phrase based on probabilities derived from their training data. This lack of genuine comprehension can result in outputs that sound plausible but are incorrect. The AI is not discerning truth from falsehood; it’s simply following learned patterns, which can sometimes lead to hallucinations.
- Contextual misinterpretation: AI models may struggle to interpret context accurately, especially in complex or nuanced situations. Without a clear understanding of the surrounding context, the AI might generate responses that are out of sync with reality, further contributing to the phenomenon of hallucination.
How to spot generative AI hallucination
Detecting AI hallucination can be challenging, especially when the outputs seem polished and credible. However, with the right approach and tools, marketers can identify and address these inaccuracies before they cause any harm. Here are some strategies to help spot AI hallucination:
- Cross-check information: One of the simplest ways to detect AI hallucination is to cross-check the AI-generated content against reliable sources. If the AI produces a statistic, fact, or claim, verify it by consulting trusted references. This step is crucial for ensuring that the information is accurate and not a result of hallucination.
- Look for unusual patterns or inconsistencies: AI hallucination often manifests as inconsistencies or patterns that don’t align with the context. For example, if the AI output includes unexpected information or shifts in tone that seem out of place, it may be a sign of hallucination. Pay close attention to any parts of the content that feel off or don’t quite fit with the rest of the message.
- Test the AI on known information: Before relying on AI for critical tasks, test it on subjects where you already know the correct answers. This can help you gauge the AI’s reliability and spot any tendencies towards hallucination. If the AI produces incorrect or misleading content in these tests, it’s a clear indicator that you need to apply more scrutiny to its outputs.
- Use AI tools designed to detect hallucination: Some advanced AI platforms include features that help identify potential hallucinations. These tools can flag content that may be questionable, allowing marketers to review and correct any inaccuracies before publication. Integrating these tools into your workflow can add an extra layer of protection against the risks of AI hallucination.
- Seek human oversight: AI should be seen as an assistant rather than a replacement for human judgement. Having a human review AI-generated content is a key step in spotting incorrect information and hallucinations. A human reviewer can apply context, experience, and critical thinking to identify and correct errors that the AI might miss.
How to prevent AI hallucination
It is essential to maintain the integrity of your marketing efforts to prevent AI hallucinations. While AI can be a powerful tool, it’s important to recognise its limitations and take proactive steps to minimise the potential for inaccuracies. Here are some strategies to help manage the risk of AI hallucination:
- Implement robust verification processes: Before deploying any AI-generated content, establish a rigorous fact-checking routine. This involves cross-referencing AI outputs with reliable sources and ensuring that any data, statistics, or claims are accurate. This step is critical to prevent the spread of misinformation and to maintain the credibility of your marketing materials.
- Select an AI system with built-in safeguards: Not all AI tools are created equal. Some platforms offer features designed to minimise the risk of hallucination, such as content validation tools that flag potentially fabricated information. When choosing an AI tool, consider those that prioritise accuracy and provide mechanisms for detecting and correcting hallucinations.
- Do your research before generating AI content: Before you prompt an AI to generate content, provide it with accurate, well-researched information. Include links or basic facts that the AI can use as a foundation. Make sure that these sources have been checked by humans for accuracy to reduce the chances of the AI producing hallucinations based on faulty data.
- Balance AI use with human oversight: While an AI algorithm can generate content quickly and efficiently, human judgment is still invaluable. Ensure that all AI-generated outputs are reviewed by a human before publication. This oversight allows for the application of context, critical thinking, and domain-specific knowledge, which can help identify and correct any hallucinations that the AI may have produced.
- Educate your team about AI limitations: Your marketing team must understand both the capabilities and the limitations of AI. Provide training on the risks associated with AI hallucination and equip your team with the skills to spot and address these issues. Awareness and education are key to preventing the unintended consequences of relying too heavily on AI.
- Develop clear guidelines for AI use: Establish internal policies that outline how AI should be used in your marketing efforts. These guidelines should specify when human intervention is required, how AI outputs should be verified, and the steps to take if hallucination is detected. Clear guidelines help ensure that AI is used responsibly and that its outputs are always subject to appropriate scrutiny.
- Train your AI: Whereas AI development might be beyond the reach of most marketers, whenever you have a chance, do train your AI to be accurate, focused on your target audience and to get its information from reliable sources.
Need support to build your AI governance?
Real-world scenarios of AI hallucination in marketing
AI hallucination is not just a theoretical concern; it could manifest in real-world marketing scenarios with significant consequences. These hypothetical scenarios highlight the importance of vigilance and careful management when using AI in marketing.
- The case of the fabricated product description: Imagine a major online retailer using AI to generate product descriptions for its vast catalogue. While the AI efficiently produces thousands of descriptions, it might start fabricating details about certain products. In one scenario, the AI could describe a simple office chair as having “massage functionality” and “ergonomic support,” neither of which are true. This could lead to customer complaints, product returns, and a dip in trust as consumers realise the descriptions are not reliable. The retailer would then need to quickly revise its content generation process to include human oversight and validation.
- False claims in AI-generated advertising: Consider an AI-driven advertising campaign for a new health supplement that goes viral for all the wrong reasons when it is discovered that the AI has generated baseless health claims. The AI, tasked with creating catchy taglines, might invent benefits like “proven to cure chronic fatigue” and “guaranteed to boost your immune system by 200%.” These claims would be entirely unfounded, leading to regulatory scrutiny and potential legal issues for the brand. This scenario would highlight the risks of relying on AI to generate content in highly regulated industries like healthcare.
- Misleading customer service: Picture a global telecom company implementing an AI chatbot to handle customer inquiries. While the bot could be effective in managing routine questions, it might hallucinate when faced with more complex issues, providing customers with incorrect billing information or even making up non-existent service plans. This could lead to customer frustration and a spike in complaints. The company might then have to intervene by retraining the AI model and increasing human oversight in customer support interactions.
- The misinterpreted data analysis: Imagine a large financial services firm using AI to generate insights for its marketing campaigns. The AI, analysing customer data, might incorrectly identify a trend suggesting that younger customers prefer premium, high-cost financial products. Based on this faulty analysis, the firm could launch a targeted campaign that completely misses the mark, resulting in poor engagement and wasted marketing spend. It might later be discovered that the AI overgeneralised a small subset of data, leading to a hallucinated conclusion that doesn’t reflect the broader customer base.
What AI tools are safer to use to avoid AI hallucination?
When considering the safety of generative AI tools, it’s important to look for platforms that prioritize transparency, accuracy, and ethical use. Here’s a list of generative AI tools that are generally regarded as safer to use, but never forget to put safety practices in place, such as the ones we listed above, to protect your content from errors.
- OpenAI’s GPT-4
- Why it’s safe: OpenAI offers is probably the world’s most used and well known generative AI model. While a level of ChatGPT hallucination could be expected, the tool offers extensive safety protocols, including content moderation tools and guidelines to prevent misuse. Users can fine-tune the model for specific applications, which increases the chance of generating accurate information and appropriate content.
- Google Cloud AI Platform
- Why it’s safe: Google’s AI tools are backed by strong safety measures, including data verification, bias detection, and explainability features. These tools help ensure that generated content is both accurate and aligned with ethical standards, and is very helpful to small businesses and startups.
- Microsoft Co-pilot
- Why it’s safe: Integrated into Microsoft 365 and enhanced by real-time search capabilities when used via Microsoft’s Edge browser or Bing Chat, Co-pilot generates content with cited sources and direct links, ensuring that information is accurate and verifiable. Microsoft also includes safeguards to prevent the generation of misleading information.
- IBM Watson
- Why it’s safe: IBM Watson emphasizes transparency and explainability, allowing users to understand how AI models reach their conclusions. Watson also includes tools for monitoring and improving model accuracy, making it a reliable option for generating content with reduced risk of hallucinations.
- Adobe Sensei
- Why it’s safe: Adobe Sensei is integrated into Adobe’s suite of creative tools and is designed with accuracy and consistency in mind. Adobe’s focus on quality control helps ensure that AI-generated content aligns with user expectations and industry standards.
- Grammarly Business
- Why it’s safe: Grammarly’s AI-powered tools are designed to assist with grammar, tone, and clarity, with a strong emphasis on correctness and reliability. Grammarly’s focus on linguistic accuracy makes it a safer choice for generating written content.
- Salesforce Einstein
- Why it’s safe: Salesforce Einstein is tailored for CRM and marketing applications, ensuring that AI-generated insights and content are based on accurate, context-specific data. Salesforce also prioritizes data privacy and provides robust tools for verifying AI outputs.
Conclusion
When used properly, generative AI models can be fantastic tools for marketers, offering unparalleled efficiency, personalisation, and data-driven insights. However, like any tool, the key to success lies in how it’s used. To mitigate AI hallucinations, make sure you implement quality control measures and retain human input to ensure that AI-generated content and strategies are not only compelling but also accurate and trustworthy.
Want to learn more about AI generated hallucinations: we recommend reading this article: How Can We Counteract Generative AI’s Hallucinations? | Digital Data Design Institute at Harvard