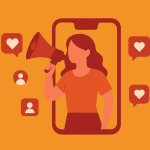
Can Data Analytics Be Replaced by AI?
With all the excitement around AI, a pressing question arises: is it on the verge of replacing data analytics as we know it? Could AI handle the intricate processes of interpreting raw data, spotting trends, and offering insights – all without human intervention? Before we jump to conclusions, let’s explore how AI is shaping the future of data analytics, if it’s likely to replace humans and whether it’s time for us to rethink its role.
Data Analytics vs. AI: Understanding the Difference
Before we dive into whether AI can replace data analytics, it’s crucial to understand what each involves.
Data analytics is the process of gathering, processing, and interpreting raw data to extract valuable insights. Analysts use various tools and techniques to identify patterns, trends, and relationships, translating numbers into actionable information that supports decision-making. While tools can assist, human expertise plays a vital role in making sense of the results and applying them to real-world contexts.
Artificial intelligence (AI), on the other hand, refers to machines designed to mimic human intelligence. AI systems can learn from data, recognise patterns, and even automate tasks. The goal of AI is to carry out complex tasks more efficiently than humans, sometimes surpassing our capacity in speed and scale.
However, the quality of AI’s results hinges heavily on how well it was trained and whether any biases or bugs are present in its algorithms. If an AI model is trained on incomplete or biased data, it can produce skewed results, leading to incorrect conclusions. Similarly, AI can make rapid calculations, but it lacks the nuanced understanding and context that humans bring to the data analytics process.
While AI is a powerful tool, it’s not a like-for-like replacement for the deep analysis, strategic thinking, and contextual awareness that human analysts bring to the table.
Before your start: Plan your AI while keeping in mind that new regulation is emerging on regular basis
As AI continues to develop, it’s not just about understanding how to integrate it into data analytics – businesses must also stay mindful of emerging regulations. In 2024, an international AI treaty was signed by the UK, EU countries and the USA, marking a major step in the global effort to ensure AI is used ethically and responsibly. This treaty outlines standards for transparency, fairness, and accountability in AI systems, with the aim of preventing misuse and harmful biases in critical sectors like finance, healthcare, and beyond.
For organisations considering AI adoption, it’s essential to factor in these new regulations. Legislation can affect how AI systems are built, trained, and deployed, particularly in how data is collected and processed. Companies will need to demonstrate that their AI models comply with local and international laws, ensuring they don’t inadvertently perpetuate biases or violate privacy standards.
This means careful planning is required when incorporating AI into data analytics workflows. You should also be flexible, able to make changes as and when they become needed to keep your AI compliant. Businesses must not only assess the technical capabilities of AI but also ensure their approach aligns with evolving legal requirements. Investing in transparency, regular audits, and working with AI systems that allow human oversight will be crucial for future-proofing your data strategy.
By staying ahead of regulations and embedding responsible AI practices, companies can leverage AI’s benefits while avoiding the risks associated with regulatory non-compliance and potential reputational damage.
Need to discuss your AI requirements?
Benefits and Limitations of AI in Data Analytics
AI technology brings significant advantages to data analytics, particularly in terms of speed, efficiency, and scale. It can process vast amounts of data in seconds, identifying trends, correlations, and anomalies that might take a human team days or even weeks to uncover. AI also excels in automation, handling repetitive tasks like data cleaning or preliminary analysis, freeing up human analysts to focus on more strategic decision-making.
However, AI also comes with its limitations. One of the biggest concerns is its reliance on the quality of training data. If the data AI learns from is incomplete, biased, or outdated, it can produce inaccurate or skewed results. Additionally, while AI can spot patterns, it lacks the contextual awareness and industry-specific knowledge needed to interpret those patterns in a meaningful way. For example, AI might flag a sudden drop in sales as an anomaly, but only a human analyst can understand the impact of external factors, like market shifts or regulatory changes, that may explain it.
Another limitation is the risk of reinforcing existing biases. AI models can inadvertently learn and amplify biases present in the data they’re trained on, leading to unfair or discriminatory outcomes, especially in areas like recruitment, lending, or policing.
While AI is a powerful tool in the analytics toolbox, it’s not a silver bullet. To maximise its benefits, AI should complement, not replace, human expertise – ensuring that results are both efficient and insightful.
The Future of AI and Data Analytics: Collaboration, Not Replacement
As AI continues to evolve, the future of data analytics is unlikely to be a story of AI replacing humans. Instead, the most promising path forward is collaboration. AI algorithms can process massive datasets, perform repetitive tasks, and identify patterns with incredible speed, but they lack the human ability that data professionals offer in terms of interpreting data in a nuanced, context-driven way.
Human analysts, with their emotional intelligence, industry expertise and ability to think critically, play a crucial role in understanding the ‘why’ behind the data. While AI might detect a trend or anomaly, it’s the analyst who considers external factors, such as market conditions or shifts in consumer behaviour, to determine what the data really means. This human insight is key to making informed, strategic decisions.
Moreover, the integration of AI into data analytics is already unlocking new possibilities. Tools that combine AI-driven automation with human oversight are helping businesses make faster, more accurate decisions. Analysts can focus on high-level analysis and creative problem-solving while leaving time-consuming tasks to AI.
By embracing this collaborative approach, organisations can leverage the strengths of both AI and human expertise. This combination allows for deeper insights, more efficient processes, and ultimately, better outcomes.
In short, the future of data analytics isn’t about AI taking over but rather about AI and human analysts working together to achieve more than either could alone.
How to Resource Your Business to Use AI
Integrating AI capabilities into your business doesn’t have to be overwhelming, but it does require careful planning. Whether you’re a small business or a large enterprise, adopting AI can enhance your data analytics efforts and boost decision-making capabilities. Here’s how to get started, depending on your business size and resources.
AI For Medium Sized or Large Businesses: Hiring and Building AI Expertise
Larger businesses have the advantage of being able to invest in skilled professionals who can fully integrate AI into the company’s data strategy. The first step is to hire a team that includes both AI specialists and data analysts. These experts will work together to build a custom AI solution tailored to your specific business needs. Whether it’s predicting customer behaviour, optimising supply chains, or enhancing marketing efforts, a dedicated team can ensure you’re making the most of AI’s potential.
It’s also important to invest in the right infrastructure – cloud services, advanced analytics platforms, and scalable data storage solutions. Combining human expertise with robust AI tools will allow your business to unlock deeper insights, utilise your historical data and maintain a competitive edge in your industry.
AI For Smaller Businesses: Affordable AI SaaS Solutions
If your business doesn’t have the resources to hire AI or data analytics experts, don’t worry. There are many user-friendly AI analytics tools and AI applications on the market that allow you to harness the power of AI without needing specialised knowledge. Platforms like Google Cloud’s AutoML, Microsoft’s Power BI, and Tableau offer intuitive interfaces where small businesses can use AI-driven insights to track trends, forecast demand, and make data-driven decisions.
For CRM and sales optimisation, tools like HubSpot AI and Salesforce Einstein can be game-changers for smaller businesses. HubSpot’s AI-powered features help you automate tasks, better understand customer data, personalise customer interactions through generative AI, and predict trends, while Salesforce Einstein uses AI to provide business intelligence and insights and recommendations for improving customer relationships and driving sales growth.
Additionally, AI tools like Zoho Analytics and MonkeyLearn are designed for businesses on a budget, allowing you to automate data analysis and gather actionable insights with minimal effort. These platforms offer comprehensive support and tutorials, making it easier to get started, even without technical expertise. By choosing the right tools, smaller businesses can still gain the benefits of AI without the need for expensive in-house experts.
Need extra resources to leverage AI?
Preparing for AI-Driven Analytics: How to Manage Your Data
Effective data management is the foundation of successful AI-driven analytics. To make the most of AI, whether it’s machine learning algorithms or generative AI models, businesses need to ensure their data is well-organised, clean, and ready for analysis. Here’s how to get started.
1. Data Management and Organisation
Good data management begins with organising your data in a structured, accessible way. Ensure you have a centralised system where all relevant data is stored, whether through cloud-based platforms or on-premise solutions. Data silos can limit the effectiveness of AI, so integrating datasets from different departments into a unified system is crucial.
2. Data Preparation: Clean and Curate
For AI models to deliver accurate results, your data needs to be clean and well-prepared. This involves removing duplicates, filling in missing values, and ensuring data is consistent across all sources. Poor data quality can lead to misleading results, especially when training machine learning algorithms or generative AI models, as they rely on accurate, well-curated data to produce meaningful insights.
3. Train AI with Relevant Data
When implementing machine learning algorithms, it’s essential to feed the models with relevant, high-quality data. The more accurate and comprehensive your data, the better your AI will perform. For generative AI models, make sure your data reflects the context and environment in which the model will operate, ensuring that it generates useful, actionable insights.
4. Maintain and Monitor
AI models aren’t a one and done solution. Continuously monitor their performance and update them as new data becomes available. Regular maintenance of your data pipeline and periodic updates to your AI models will ensure that your analytics remain relevant and reliable over time.
What Type of Freelancers or Employees Should You Hire to Make the Best of AI Capabilities?
When integrating AI into your business, hiring the right talent is crucial. From data scientists to data engineers, each role contributes uniquely to your AI-driven data analytics strategy. Here’s a guide to help you understand what types of professionals you should look for.
1. Data Analysts and Data Scientists: A Crucial Partnership
Both data analysts and data scientists play vital roles in maximising AI’s capabilities. A data analyst focuses on traditional analytics, interpreting trends, generating reports, and ensuring data quality. While there’s talk about “AI replacing data analysts,” human analysts remain essential for providing contextual understanding and domain expertise that AI cannot replicate.
Meanwhile, a data scientist brings advanced skills in predictive analytics, machine learning, and AI. They build and optimise models, using AI and generative AI techniques to forecast trends and extract deeper insights from complex datasets. Together, analysts and data scientists can transform your data strategy and help you stay ahead in a competitive market.
2. Data Engineers: Building AI Infrastructure
A data engineer is key to managing the infrastructure that supports your AI systems. They ensure your data is clean, well-organised, and accessible, so it can be effectively used by AI models. By building and maintaining data pipelines, data engineers ensure that both data analysts and AI data analysts have access to high-quality, reliable data.
Hiring a skilled data engineer can be critical to the success of your AI initiatives, as they ensure that all systems run smoothly, feeding accurate data into both traditional analytics and AI systems.
3. AI Experts: Unlocking the Potential of Gen AI
For businesses looking to push the boundaries of AI, hiring an expert in generative AI (Gen AI) and machine learning can be transformative. These professionals specialise in training AI systems and optimising them for tasks like predictive analytics, content generation, and automated decision-making. While data analysts and scientists manage the day-to-day data analytics jobs, generative AI experts focus on building systems that automate advanced processes and push the limits of what AI can achieve.
Want a human-to-human conversation about your AI requirements?